RiskSpan Edge & CRT Data
For participants in the credit risk transfer (CRT) market, managing the massive quantity of data to produce clear insights into deal performance can be difficult and demanding on legacy systems. Complete analysis of the deals involves bringing together historical data, predictive models, and deal cash flow logic, often leading to a complex workflow in multiple systems. RiskSpan’s Edge platform (RS Edge) solves these challenges, bringing together all aspects of CRT analysis. RiskSpan is the only vendor to bring together everything a CRT analyst needs:
- Normalized, clean, enhanced data across programs (STACR/CAS/ACIS/CIRT),
- Historical Fannie/Freddie performance data normalized to a single standard,
- Ability to load loan-level files related to private risk transfer deals,
- An Agency-specific, loan-level, credit model,
- Seamless Intex integration for deal and portfolio analysis,
- Scalable scenario analysis at the deal or portfolio level, and
- Vendor and client model integration capabilities.
- Ability to load loan-level files related to private risk transfer deals.
All of these features are built into RS Edge, a cloud-native, data and analytics platform for loans and securities. The RS Edge user interface is accessible via any web browser, and the processing engine is accessible via an application programming interface (API). Accessing RS Edge via the API allows access to the full functionality of the platform, with direct integration into existing workflows in legacy systems such as Excel, Python, and R. To tailor RS Edge to the specific needs of a CRT investor, RiskSpan is rolling out a series of Excel tools, built using our APIs, which allow for powerful loan-level analysis from the tool everyone knows and loves. Accessing RS Edge via our new Excel templates, users can:
- Track deal performance,
- Compare deal profiles,
- Research historical performance of the full GSE population,
- Project deal and portfolio performance with our Agency-specific credit model or with user-defined CPR/CDR/severity vectors, and
- Analyze various macro scenarios across deals or a full portfolio
The web-based user interface allows for on-demand analytics, giving users specific insights on deals as the needs arise. The Excel template built with our API allows for a targeted view tailored to the specific needs of a CRT investor.
For teams that prefer to focus their time on outcomes rather than the build, RiskSpan’s data team can build custom templates around specific customer processes. RiskSpan offers support from premiere data scientists who work with clients to understand their unique concerns and objectives to integrate our analytics with their legacy system of choice. The images are examples of a RiskSpan template for CRT deal comparison: profile comparison, loan credit score distribution, and delinquency performance for five Agency credit risk transfer deals, pulled via the RiskSpan Data API and rendered in Excel. ______________________________________________________________________________________________
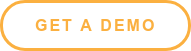